Mastering Video Annotation Tools in Computer Vision
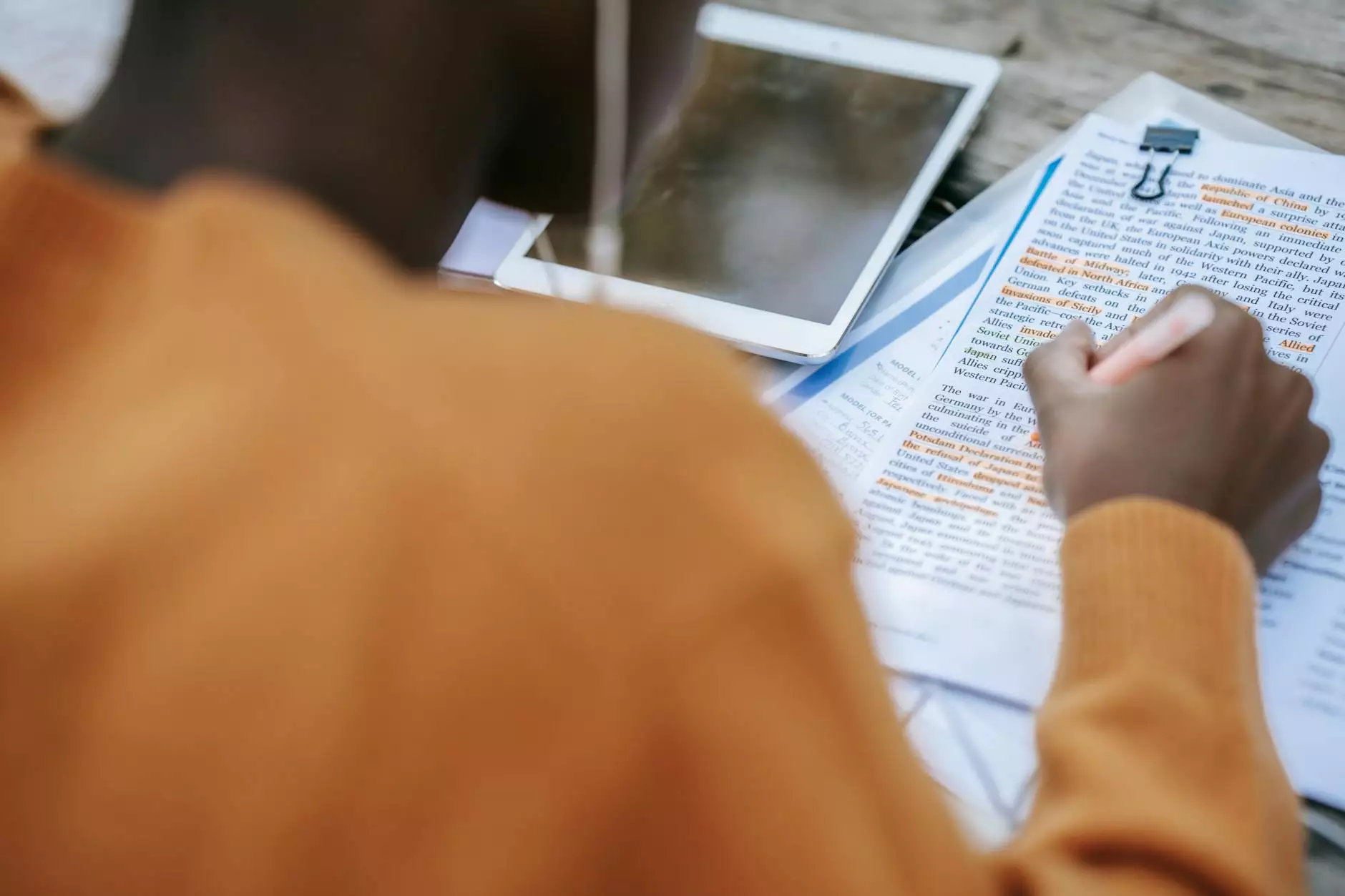
In today's digital landscape, the role of video annotation tools within the realm of computer vision is proving to be indispensable. These tools are pivotal in transforming raw video footage into structured data sets that power machine learning algorithms, fostering advancements in various fields such as autonomous driving, surveillance, and content moderation. This article delves into the intricacies of video annotation tools, their functionalities, and the myriad benefits they offer to businesses leveraging data annotation platforms like those provided by KeyLabs.ai.
Understanding Video Annotation
Video annotation is the process of adding descriptive labels to different elements within video data. This task is crucial for training computer vision models, enabling machines to interpret and understand video content just like humans do. The objectives of video annotation typically include:
- Object Detection: Identifying and classifying objects within each frame.
- Tracking: Following the movement of objects across frames.
- Segmentation: Outlining or cropping specific areas in a video, often for focused analysis.
- Event Detection: Recognizing and labeling specific events or actions in the video sequence.
The Importance of a Video Annotation Tool
With the increasing volume of video data produced each day, the demand for efficient annotation tools has surged. Businesses are now relying on specialized video annotation tools to streamline their workflow. Here’s why they are essential:
1. Enhanced Accuracy
Video annotation tools come equipped with features that enhance accuracy in data labeling. By allowing professionals to adjust labels frame-by-frame, companies can ensure higher precision in their datasets, which is crucial for training effective models.
2. Improved Efficiency
Manual annotation is often time-consuming. Automated features in video annotation tools significantly reduce the time required to annotate large datasets. For instance, tools can automatically generate predictions which annotators can then verify, speeding up the overall process.
3. Scalability
For businesses scaling their operations, video annotation tools provide the scalability needed to handle increasing amounts of data. Whether it involves annotating thousands of hours of footage or expanding to new projects, these tools offer the flexibility and resources necessary for growth.
Key Features of Video Annotation Tools
For businesses considering a video annotation tool for computer vision projects, it’s crucial to evaluate several key features:1. User-Friendly Interface
A user-friendly interface simplifies the annotation process, especially for teams that may not have a strong technical background. Tools should provide intuitive controls and straightforward workflows to ensure that annotators can work effectively without extensive training.
2. Various Annotation Types
Look for tools that support different types of annotations:
- Bounding Boxes: Used for object detection tasks.
- Polygons: Ideal for more complex object shapes.
- Keypoints: Useful for tasks like pose estimation.
- Semantic Segmentation: For pixel-wise classification.
3. Collaboration Features
Effective collaboration tools facilitate teamwork, making it easier for multiple annotators to work on a project simultaneously. Features such as task assignment, feedback loops, and progress tracking are essential for ensuring that projects stay on track.
4. Integration with Other Platforms
Seamless integration with data management systems or machine learning frameworks is vital. This enables businesses to easily transition from the annotation phase to the model training phase. Tools that support APIs or direct connections to popular machine learning platforms will enhance the overall workflow.
5. Quality Assurance Mechanisms
Built-in quality assurance processes can significantly improve the reliability of annotations. Look for tools that offer multi-tier review systems where annotations can be double-checked for accuracy and consistency.
Choosing the Right Video Annotation Tool
Selecting the right video annotation tool involves careful consideration of your project needs. Here are some tips for making the right choice:1. Assess Your Requirements
Evaluate the specific needs of your project. Are you focusing on object detection, activity recognition, or some other aspect of video analysis? Different projects may call for different functionalities in an annotation tool.
2. Explore Available Options
Research various video annotation tools available in the market. Many platforms offer free trials, allowing you to test functionalities before making a commitment.
3. Consider Budget Constraints
Video annotation tools can vary significantly in pricing. Assess your budget and look for tools that offer the best value for your specific requirements.
4. Read User Reviews
User reviews and case studies can provide valuable insights into the strengths and weaknesses of different annotation tools. Check reputable sources and consider both positive and negative feedback.
5. Look for Customer Support
Robust customer support can make a huge difference, especially when encountering issues during the annotation process. Choose tools that provide comprehensive support, including documentation, tutorials, and direct help from staff.
Implementation Strategies for Successful Video Annotation Projects
Once you’ve selected a video annotation tool, the next step involves strategically implementing it within your operations. Here are some effective strategies to ensure success:1. Training Your Team
Invest time in properly training your annotation team on the chosen tool. A well-trained team will be more efficient and produce higher-quality annotations.
2. Define Clear Guidelines
Establishing clear guidelines for annotation creates uniformity, reducing discrepancies between annotators. This standardization is key to producing reliable datasets.
3. Regularly Review Annotations
Incorporate regular reviews of annotated data to maintain quality. Feedback loops and iterative improvements can lead to burgeoning accuracy over time.
4. Encourage Collaboration and Communication
Foster a culture of collaboration among your team. Open communication about challenges faced during the annotation process can lead to innovative solutions and enhancements in your methodology.
5. Start with Pilot Projects
To mitigate risks, start with smaller pilot projects before scaling to larger datasets. This approach allows you to test processes and tools effectively, making adjustments as necessary.
Case Studies: Successful Implementation of Video Annotation Tools
Examining real-world applications of video annotation tools can provide invaluable insights. Below, we highlight a few success stories from various industries:
1. Autonomous Vehicles
Companies like Tesla and Waymo have successfully utilized video annotation tools to label vast amounts of driving footage, facilitating the training of their advanced driver-assistance systems (ADAS). These annotations help vehicles detect pedestrians, cyclists, and other vehicles, enhancing safety and reliability on the roads.
2. Security and Surveillance
In the realm of security, organizations leverage video annotation for refining face recognition systems and identifying suspicious activities. By labeling video feeds from surveillance cameras, companies can train their systems to better understand and react to potential threats.
3. Sports Analytics
Sports teams are increasingly using video annotation to analyze player performances and game strategies. Annotated video footage allows teams to break down gameplay, identifying strengths and weaknesses, thus enhancing training effectiveness.
4. Medical Imaging
In healthcare, video annotation is being employed to assess patient movements in physiotherapy or rehabilitation contexts. This technology aids practitioners in monitoring progress and adjusting treatment plans based on data-driven insights.
The Future of Video Annotation in Computer Vision
The evolution of technology means that the future holds exciting possibilities for video annotation in computer vision. With advancements in artificial intelligence and machine learning, we can expect:
- Increased Automation: As AI algorithms become more sophisticated, the degree of automation within annotation tools will reduce the reliance on human input.
- Improved Accuracy: Innovations in computer vision technology are set to enhance the accuracy of both automated and manual annotations.
- Greater Integration: Future tools will likely see broader integration capabilities with various platforms and services, allowing for more seamless workflows.
- Accessibility of Tools: Video annotation tools will become more user-friendly and accessible, enabling smaller teams and businesses to leverage this essential technology efficiently.
Conclusion
As video data continues to burgeon across industries, the importance of effective video annotation tools in computer vision cannot be overstated. Utilizing these tools effectively can lead to breakthroughs in technology, improve workflows, and drive success in data-driven initiatives. Organizations like KeyLabs.ai are at the forefront of providing innovative data annotation platforms that equip teams with the capabilities to tackle the challenges of video data annotation head-on. Embracing these tools paves the way for advancements across numerous sectors, making it an exciting time for businesses engaged in computer vision projects.
video annotation tool computer vision