The Impact of Machine Learning on Modern Business Practices
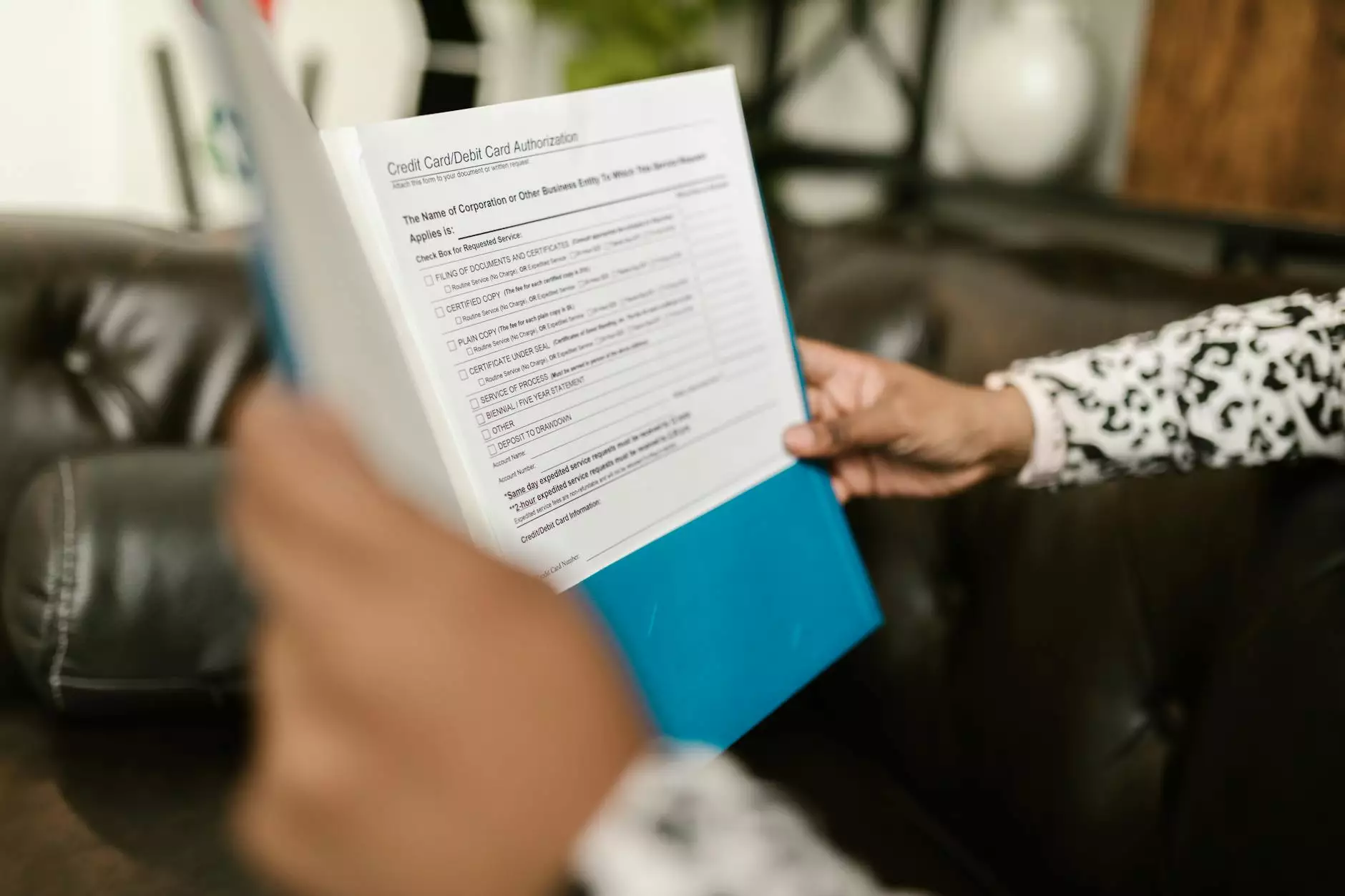
In today’s rapidly evolving business landscape, the integration of machine learning has become a crucial element for organizations seeking to enhance efficiency, decision-making, and customer engagement. As industries worldwide embrace new technologies, machine learning emerges as a transformative force, reshaping traditional practices and paving the way for innovative strategies.
Understanding Machine Learning
Machine learning, a subset of artificial intelligence, refers to the capability of systems to learn from data, identify patterns, and make decisions with minimal human intervention. By utilizing algorithms, machine learning enables organizations to analyze vast amounts of data, turning this data into actionable insights.
What Are the Key Components of Machine Learning?
- Data: The foundation of machine learning. More data typically leads to more accurate models.
- Algorithms: Mathematical models that process the data and learn from it.
- Computational Power: The capability to process complex calculations and large datasets quickly.
- Feedback Mechanisms: Systems that allow machine learning models to adjust and improve based on new data and outcomes.
Revolutionizing Business Operations
The influence of machine learning extends across various business sectors, enhancing operations drastically. Here are several ways it is being utilized:
1. Improved Decision Making
Businesses can leverage machine learning algorithms to process customer data, market trends, and operational metrics, leading to more informed decisions. By analyzing patterns in data, organizations can identify areas for improvement and capitalize on emerging opportunities.
2. Enhanced Customer Experience
Tailoring experiences to meet customer expectations is paramount. Machine learning algorithms analyze customer behaviors and preferences, allowing businesses to offer personalized recommendations, targeted marketing campaigns, and improved customer support.
- Recommendation Systems: Utilizing data to suggest products or services that match customer preferences.
- Chatbots: Machine learning powers intelligent chatbots that provide 24/7 customer service and support.
3. Predictive Analytics
Predictive analytics, driven by machine learning, enables businesses to forecast future outcomes based on historical data. This capability is invaluable in various domains:
- Inventory Management: Anticipating demand to optimize stock levels and reduce costs.
- Financial Analysis: Predicting market trends and investment opportunities.
- Risk Management: Identifying potential risks and developing mitigation strategies.
Machine Learning Applications Across Industries
The versatility of machine learning allows its application across a myriad of industries:
Healthcare
In the healthcare sector, machine learning enhances diagnostics, treatment protocols, and operational efficiencies. For example:
- Medical Imaging: Algorithms analyze medical images to detect anomalies like tumors or fractures with high accuracy.
- Predictive Healthcare: Algorithms can predict disease outbreaks and patient admissions by analyzing various data sources.
Finance
The finance industry utilizes machine learning for fraud detection, algorithmic trading, and credit scoring:
- Fraud Detection: Systems learn from transaction data to identify unusual patterns that may indicate fraudulent activities.
- Automated Trading: Algorithms execute trades based on predictive analytics derived from market data.
Retail
In retail, machine learning optimizes supply chains, enhances customer experiences, and boosts sales strategies. Examples include:
- Dynamic Pricing: Algorithms analyze market conditions to adjust prices in real-time for competitive advantage.
- Inventory Optimization: Predictive analytics forecast demand, helping retailers manage stock levels effectively.
Challenges in Implementing Machine Learning
Despite its potential, implementing machine learning is not without challenges. Organizations face several hurdles, including:
Data Quality and Availability
The success of machine learning models heavily relies on the quality and quantity of data. Poor-quality or insufficient data can lead to inaccurate predictions and misinformed decisions.
Skill Gaps
Finding skilled professionals who understand machine learning algorithms and their applications can be a challenge for many businesses. Continuous training and development are crucial to keep up with technological advancements.
Integration with Existing Systems
Integrating machine learning solutions into existing workflows and systems can be complex and time-consuming, requiring careful planning and testing.
Strategies for Successful Machine Learning Adoption
To successfully implement machine learning in your business, consider the following strategies:
1. Invest in Data Infrastructure
Ensure you have the right data infrastructure in place. This includes robust data collection, storage, and processing capabilities. High-quality data pipelines are essential for feeding machine learning algorithms.
2. Foster a Data-Driven Culture
Encouraging a culture that values data and insights will promote the adoption of machine learning initiatives. Educate employees on the benefits of data-driven decision-making.
3. Collaborate with Experts
Engaging with machine learning consultants or creating partnerships with tech firms can expedite your learning curve and implementation process.
4. Start Small
Begin with pilot projects to test machine learning applications, assessing their impact and scalability before a full rollout.
The Future of Machine Learning in Business
As machine learning technology continues to advance, its implications for businesses are vast. Anticipated trends include:
- Increased Automation: Machine learning will automate more tasks, freeing up human resources for strategic initiatives.
- Greater Personalization: Customer experiences will continue to be more tailored, leveraging extensive data insights.
- Ethical Considerations: The focus on ethical AI practices will grow, necessitating transparency and fairness in machine learning applications.
Conclusion
In conclusion, the impact of machine learning on business is profound and far-reaching. As organizations worldwide embrace this technology, they unlock new levels of efficiency, customer engagement, and innovative capabilities. By understanding its applications, challenges, and strategies for implementation, businesses can position themselves at the forefront of industry evolution, leveraging the full potential of machine learning to drive growth and success.
machine learning article